Effective Ways to Calculate Uncertainty in 2025: Learn More
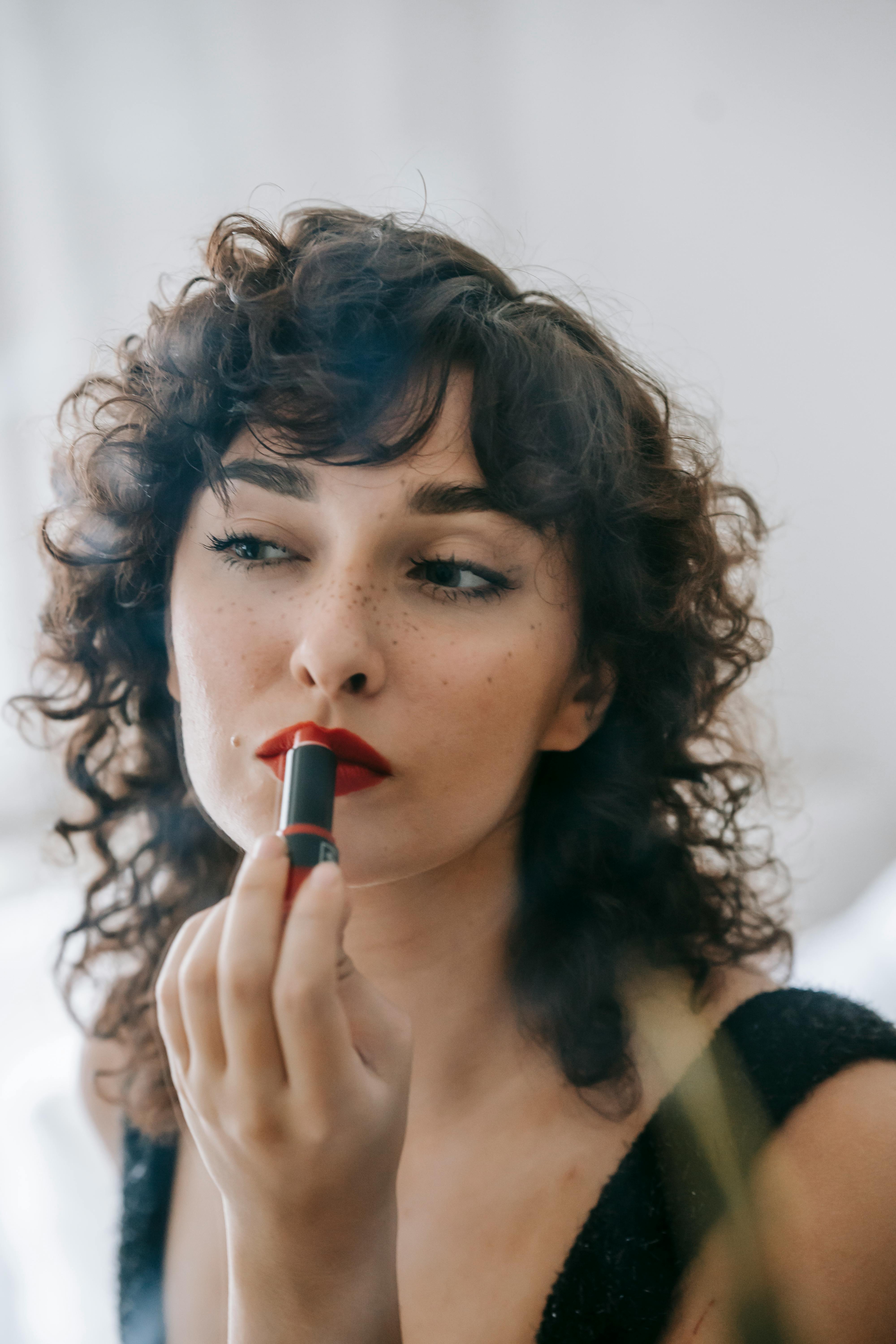
Effective Ways to Calculate Uncertainty in 2025: Learn More
In the realm of science, mathematics, engineering, and beyond, understanding and calculating uncertainty is critical for making informed decisions. As we delve into 2025, the complexity of uncertainty becomes more pronounced, presenting both challenges and opportunities for researchers and professionals alike. This article aims to explore the various effective methods of calculating uncertainty, the sources and types of uncertainty, and how they intersect with statistical practices and real-world applications.
Understanding uncertainty is essential, as it influences decisions, predictions, and assessments across various domains. Whether one is handling uncertainty in research or engaging in uncertainty quantification for statistical analysis, grasping these concepts can significantly enhance accuracy and reliability. In this article, readers will discover key principles for calculating uncertainty and effective tools that facilitate precision in measurement and data interpretation.
We will navigate the extensive landscape of uncertainty—from basic definitions to advanced frameworks. Key takeaways include understanding different sources of uncertainty, evaluating uncertainty in outcomes, and implementing strategies for minimizing uncertainty. As you read along, you will find practical examples and insights that can empower your work in handling uncertainty effectively.
Understanding the Types of Uncertainty
Building on our foundational overview, it's crucial to categorize the various types of uncertainty encountered in scientific inquiries and data analysis. Broadly, uncertainties can be classified into systematic and random uncertainties. Systematic uncertainties arise from consistent biases in measurement processes, while random uncertainties are the result of variability in data collection and experimental conditions.
Systematic Uncertainties
Systematic uncertainties often stem from calibration errors or environmental factors that consistently affect measurements. For instance, a poorly calibrated scale will consistently report incorrect weights, leading to systematic errors in data interpretation. Understanding these uncertainties is vital for developing methodologies to eliminate or reduce their impact.
Random Uncertainties
In contrast, random uncertainties are typically unpredictable and arise from inherent variability in both measurement processes and the objects being measured. These uncertainties can often be quantified through statistical methods, helping researchers to estimate the confidence intervals of their data. It is essential to incorporate these uncertainty assessments for valid interpretations of experimental results.
Measurement Uncertainties
Measurement uncertainty can take various forms, including instrumental, environmental, and methodological uncertainties. For researchers, it's essential to identify these uncertainties early in the design phase of experiments to ensure reliable data collection and analysis. Understanding measurement uncertainty contributes towards effective uncertainty resolution and enhances confidence in the reported outcomes.
With a grasp on the types of uncertainty, we can now explore methods for calculating and evaluating uncertainty in practice.
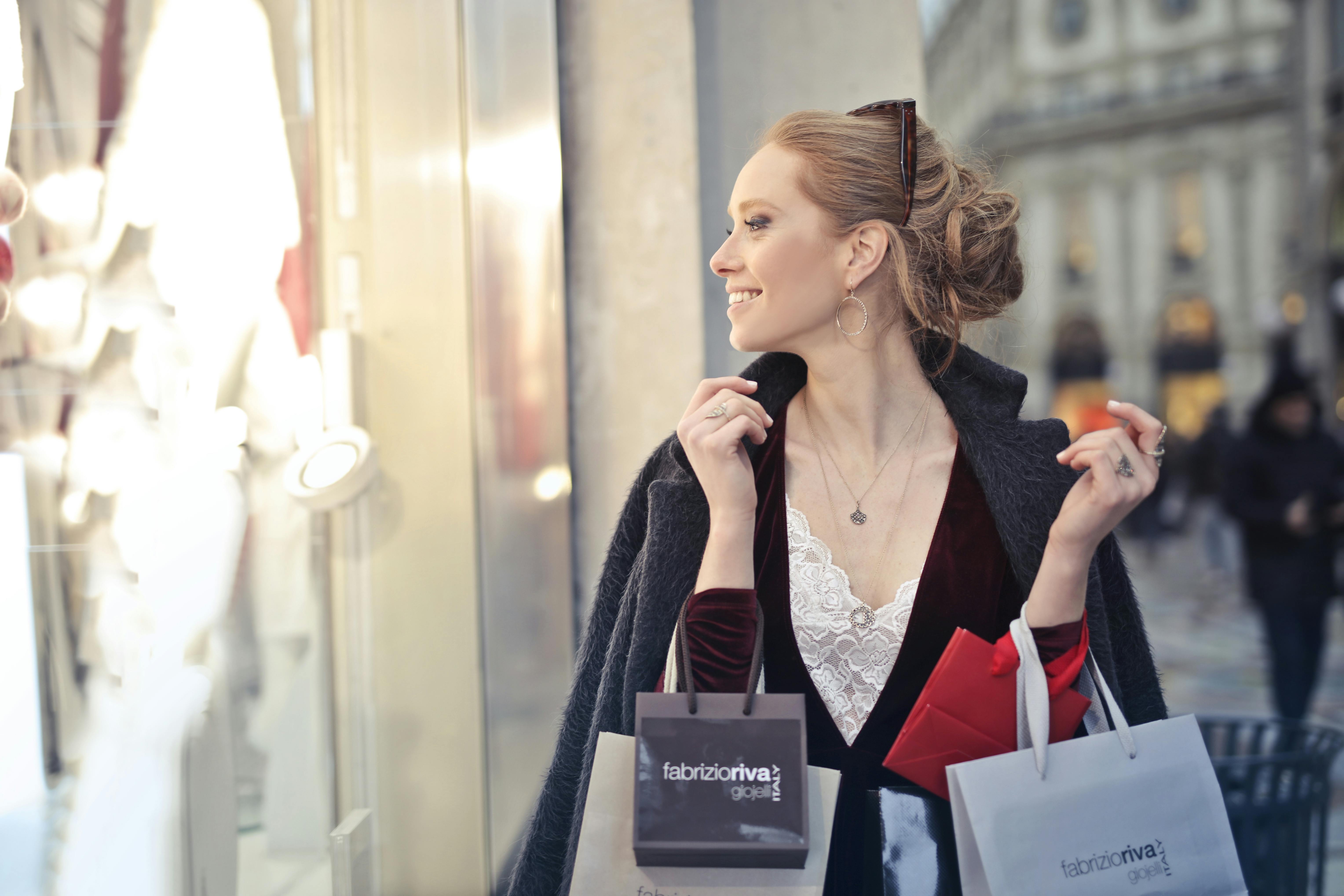
Methods for Calculating Uncertainty
Now that we have differentiated between types of uncertainty, the next logical step is understanding how to accurately calculate it. Various methods exist, each tailored to specific types of data and experimental conditions. These methods are crucial for ensuring that uncertainty is adequately quantified and communicated.
Basic Uncertainty Formula
The basic uncertainty formula serves as the foundation for calculating uncertainties in measurements. By applying statistical principles, this formula helps quantify the effects of different uncertainty components. Generally, the formula is expressed as:
U = sqrt(U12 + U22 + ... + Un2)
This formula demonstrates that the combined uncertainty (U) is derived from the root sum of squares of individual uncertainties (U1, U2, ..., Un). Implementing this approach equips researchers with a systematic way to estimate uncertainties in their experiments.
Error Propagation
Error propagation is a technique used to analyze how uncertainties in individual measurements contribute to overall uncertainty in a derived quantity. This method often employs calculus, helping in identifying which sources of uncertainty have the most significant impact. It's particularly useful in enhancing our understanding of uncertainty propagation, especially in complex calculations involving multiple variables.
Monte Carlo Simulation
Another prominent method is the Monte Carlo simulation, which utilizes random sampling to analyze uncertainty. This method allows for the comprehensive assessment of uncertainty by generating a wide range of possible outcomes based on input variables' uncertainty. By simulating numerous scenarios, researchers can visualize uncertainty distributions and make informed predictions about real-world outcomes.
As we transition into practical applications of these calculations, it is essential to explore the sources of uncertainty that researchers might encounter in their specific fields.
Sources of Uncertainty in Research
Identifying sources of uncertainty is a critical step in accurately assessing uncertainty in any research study. Generally, sources of uncertainty can be divided into three categories: measurement uncertainties, methodological uncertainties, and external factors.
Measurement Uncertainties
Measurement uncertainties are directly tied to the equipment and techniques used during data collection. These can include calibration errors, sensor limitations, or manual reading errors. Ensuring that measurement instruments are precisely calibrated and maintained is fundamental to minimizing these uncertainties.
Methodological Uncertainties
Methodological uncertainties refer to the design and execution of the research study. Choices regarding sampling methods, experimental protocols, and data analysis techniques can introduce uncertainties. Incorporating best practices and guidelines for uncertainty evaluation throughout the research process can mitigate these issues.
External Factors
External factors, such as environmental conditions or human errors, also contribute significantly to uncertainty. Acknowledging these factors allows researchers to implement potential controls and understand their influence on the results. Developing resilience to external fluctuations can ultimately bolster the reliability of experimental outcomes.
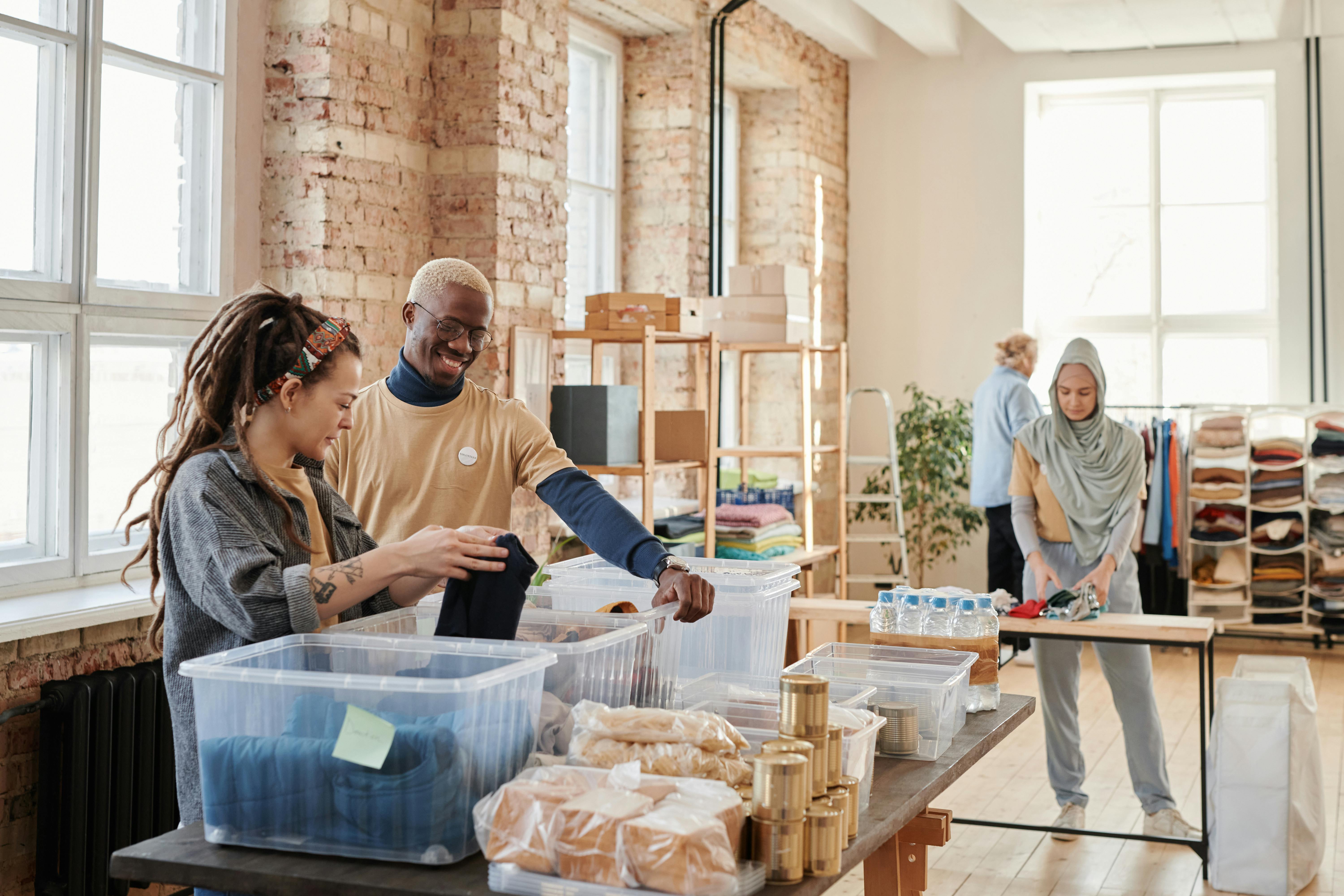
Reducing Uncertainty in Measurements
With a deeper understanding of the various sources of uncertainty, the next focus should be on effective techniques for reducing uncertainty in measurements and analyses. Implementing effective uncertainty reduction techniques can significantly enhance the reliability of findings in any research context.
Calibration and Maintenance
Regular calibration and proper maintenance of measurement instruments are imperative in minimizing systematic uncertainties. Standardizing those processes according to established protocols can ensure that instruments remain accurate, thereby reducing measurement errors.
Improved Methodologies
Utilizing advanced methodologies tailored to the specific fields of study can also significantly reduce uncertainty. For example, employing better sampling methods or selecting appropriate statistical techniques allows researchers to collect more precise data. Careful consideration of experimental design helps in identifying potential weaknesses that could lead to uncertainties.
Training and Expertise
Investing in training researchers in uncertainty principles and error analysis is essential. Ensuring that personnel are well-equipped to recognize and handle uncertainties contributes to better decision-making and confidence in reported results. Engagement with expert communities can also promote the sharing of best practices and innovative solutions for managing uncertainty.
Q&A: Common Questions about Uncertainty
What are the common sources of uncertainty?
Common sources of uncertainty include measurement uncertainties, methodological uncertainties, and environmental factors that may affect the research results. For example, systematic biases in measurements or uncontrolled environmental variables could introduce significant uncertainties.
How can I effectively calculate uncertainty in my research?
To calculate uncertainty, it's recommended to use established methods such as the standard uncertainty formula, error propagation techniques, or Monte Carlo simulations. These methods can enhance the robustness of your uncertainty assessments.
Why is understanding uncertainty important in research?
Understanding uncertainty is critical as it influences decision-making, predictions, and the credibility of conclusions drawn from research. Quantifying uncertainty properly enables researchers to communicate the reliability of their findings effectively.
This exploration of effective ways to calculate uncertainty in scientific research is integral to advancing accuracy and reliability. As uncertainty frameworks continue to evolve and expand, implementing these strategies will be pivotal in addressing the inherent uncertainties across various fields.